Live Session
Wednesday Posters
Main Track
A Probabilistic Position Bias Model for Short-Video Recommendation Feeds
Olivier Jeunen (ShareChat)
Abstract
Modern web-based platforms often show ranked lists of recommendations to users, in an attempt to maximise user satisfaction or business metrics. Typically, the goal of such systems boils down to maximising the exposure probability — conversely, minimising the rank— for items that are deemed “reward-maximising” according to some metric of interest. This general framing comprises music or movie streaming applications, as well as e-commerce, restaurant or job recommendations, and even web search. Position bias or user models can be used to estimate exposure probabilities for each use-case, specifically tailored to how users interact with the presented rankings. A unifying factor in these diverse problem settings is that typically only one or several items will be engaged with (clicked, streamed, purchased, et cetera) before a user leaves the ranked list. Short-video feeds on social media platforms diverge from this general framing in several ways, most notably that users do not tend to leave the feed after, for example, liking a post. Indeed, seemingly infinite feeds invite users to scroll further down the ranked list. For this reason, existing position bias or user models tend to fall short in such settings, as they do not accurately capture users’ interaction modalities. In this work, we propose a novel and probabilistically sound personalised position bias model for feed recommendations. We focus on a 1st-level feed in a hierarchical structure, where users may enter a 2nd-level feed via any given 1st-level item. We posit that users come to the platform with a given scrolling budget that is drawn according to a discrete power-law distribution, and show how the survival function of said distribution can be used to obtain closed-form estimates for personalised exposure probabilities. Empirical insights gained through data from a large-scale social media platform show how our probabilistic position bias model more accurately captures empirical exposure than existing models, and paves the way for improved unbiased evaluation and learning-to-rank.
Join the Conversation
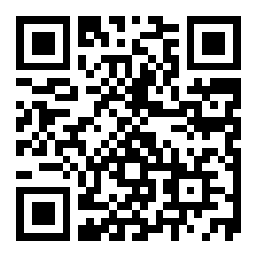