Live Session
Wednesday Posters
Main Track
ADRNet: A Generalized Collaborative Filtering Framework Combining Clinical and Non-Clinical Data for Adverse Drug Reaction Prediction
Haoxuan Li (Center for Data Science, Peking University), Taojun Hu (Peking University), Zetong Xiong (Zhongnan University of Economic and Law), Chunyuan Zheng (University of California, San Diego), Fuli Feng (University of Science and Technology of China), Xiangnan He (University of Science and Technology of China) and Xiao-Hua Zhou (Peking University)
Abstract
Adverse drug reaction (ADR) prediction plays a crucial role in both health care and drug discovery for reducing patient mortality and enhancing drug safety. Recently, many studies have been devoted to effectively predict the drug-ADRs incidence rates. However, these methods either did not effectively utilize non-clinical data, i.e., physical, chemical, and biological information about the drug, or did little to establish a link between content-based and pure collaborative filtering during the training phase. In this paper, we first formulate the prediction of multi-label ADRs as a drug-ADR collaborative filtering problem, and to the best of our knowledge, this is the first work to provide extensive benchmark results of previous collaborative filtering methods on two large publicly available clinical datasets. Then, by exploiting the easy accessible drug characteristics from non-clinical data, we propose ADRNet, a generalized collaborative filtering framework combining clinical and non-clinical data for drug-ADR prediction. Specifically, ADRNet has a shallow collaborative filtering module and a deep drug representation module, which can exploit the high-dimensional drug descriptors to further guide the learning of low-dimensional ADR latent embeddings, which incorporates both the benefits of collaborative filtering and representation learning. Extensive experiments are conducted on two publicly available real-world drug-ADR clinical datasets and two non-clinical datasets to demonstrate the accuracy and efficiency of the proposed ADRNet.
Join the Conversation
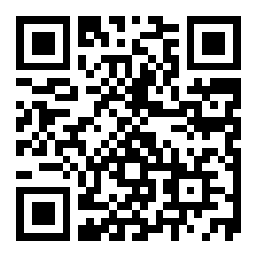