Live Session
Session 7: Interactive Recommendation 1
Research
Alleviating the Long-Tail Problem in Conversational Recommender Systems
Zhipeng Zhao (Singapore Management University), Kun Zhou (School of Information, Renmin University of China), Xiaolei Wang (Gaoling School of Artificial Intelligence, Renmin University of China), Wayne Xin Zhao (Gaoling School of Artificial Intelligence, Renmin University of China), Fan Pan (Poisson Lab, Huawei), Zhao Cao (Poisson Lab, Huawei) and Ji-Rong Wen (Gaoling School of Artificial Intelligence, Renmin University of China)
Abstract
Conversational recommender systems (CRS) aim to provide the recommendation service via natural language conversations. To develop an effective CRS, high-quality CRS datasets are very crucial. However, existing CRS datasets suffer from the long-tail issue, \ie a large proportion of items are rarely (or even never) mentioned in the conversations, which are called long-tail items. As a result, the CRSs trained on these datasets tend to recommend frequent items, and the diversity of the recommended items would be largely reduced, making users easier to get bored.
To address this issue, this paper presents \textbf{LOT-CRS}, a novel framework that focuses on simulating and utilizing a balanced CRS dataset (\ie covering all the items evenly) for improving \textbf{LO}ng-\textbf{T}ail recommendation performance of CRSs. In our approach, we design two pre-training tasks to enhance the understanding of simulated conversation for long-tail items, and adopt retrieval-augmented fine-tuning with label smoothness strategy to further improve the recommendation of long-tail items. Extensive experiments on two public CRS datasets have demonstrated the effectiveness and extensibility of our approach, especially on long-tail recommendation. All the experimental codes will be released after the review period.
Join the Conversation
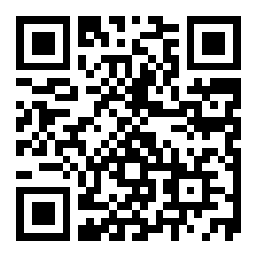