Live Session
Session 1: Collaborative filtering 1
Research
Augmented Negative Sampling for Collaborative Filtering
Yuhan Zhao (Harbin Engineering University), Rui Chen (Harbin Engineering University), Riwei Lai (Harbin Engineering University), Qilong Han (Harbin Engineering University), Hongtao Song (Harbin Engineering University) and Li Chen (Hong Kong Baptist University)
Abstract
Negative sampling is essential for implicit-feedback-based collaborative filtering, which is used to constitute negative signals from massive unlabeled data to guide supervised learning. The state-of-the-art idea is to utilize hard negative samples that carry more useful information to form a better decision boundary. To balance efficiency and effectiveness, the vast majority of existing methods follow the two-pass approach, in which the first pass samples a fixed number of unobserved items by a simple static distribution and then the second pass selects the final negative items using a more sophisticated negative sampling strategy. However, selecting negative samples from the original items from a dataset is inherently limited due to the limited available choices, and thus may not be able to contrast positive samples well. In this paper, we confirm this observation via carefully designed experiments and introduce two major limitations of existing solutions: ambiguous trap and information discrimination.
Our response to such limitations is to introduce “augmented” negative samples that may not exist in the original dataset. This direction renders a substantial technical challenge because constructing unconstrained negative samples may introduce excessive noise that eventually distorts the decision boundary. To this end, we introduce a novel generic augmented negative sampling (ANS) paradigm and provide a concrete instantiation. First, we disentangle the hard and easy factors of negative items. Next, we generate new candidate negative samples by augmenting only the easy factors in a regulated manner: the direction and magnitude of the augmentation are carefully calibrated. Finally, we design an advanced negative sampling strategy to identify the final augmented negative samples, which considers not only the score used in existing methods but also a new metric called augmentation gain. Extensive experiments on five real-world datasets demonstrate that our method significantly outperforms state-of-the-art baselines. Our code is publicly available at https://anonymous.4open.science/r/ANS-Recbole-B070/.
Join the Conversation
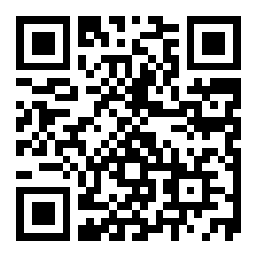