Live Session
Thursday Posters
Main Track
Co-occurrence Embedding Enhancement for Long-tail Problem in Multi-Interest Recommendation
Yaokun Liu (Tianjin University), Xiaowang Zhang (Tianjin University), Minghui Zou (Tianjin University) and Zhiyong Feng (Tianjin University).
Abstract
Multi-interest recommendation methods extract multiple interest vectors to represent the user comprehensively. Despite their success in the matching stage, previous works overlook the long-tail problem. This results in the model excelling at suggesting head items, while the performance for tail items, which make up more than 70% of all items, remains suboptimal. Hence, enhancing the tail item recommendation capability holds great potential for improving the performance of the multi-interest model.
Through experimental analysis, we reveal that the insufficient context for embedding learning is the reason behind the underperformance of tail items. Meanwhile, we face two challenges in addressing this issue: the absence of supplementary item features and the need to maintain head item performance. To tackle these challenges, we propose a CoLT module (Co-occurrence embedding enhancement for Long-Tail problem) that replaces the embedding layer of existing multi-interest frameworks. By linking co-occurring items to establish “assistance relationships”, CoLT aggregates information from relevant head items into tail item embeddings and enables joint gradient updates. Experiments on three datasets show our method outperforms SOTA models by 21.86% Recall@50 and improves the Recall@50 of tail items by 14.62% on average.
Join the Conversation
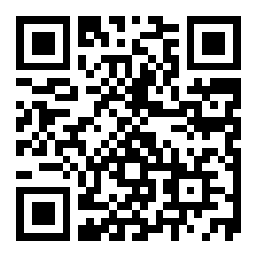