Live Session
Session 11: Sequential Recommendation 2
Research
Contrastive Learning with Frequency-Domain Interest Trends for Sequential Recommendation
Yichi Zhang (Harbin Engineering University), Guisheng Yin (Harbin Engineering University) and Yuxin Dong (Harbin Engineering University)
Abstract
Recently, contrastive learning for sequential recommendation has demonstrated its powerful ability to learn high-quality user representations. However, constructing augmented samples in the time domain poses challenges due to various reasons, such as fast-evolving trends, interest shifts, and system factors. Furthermore, the F-principle indicates that deep learning preferentially fits the low-frequency part, resulting in poor performance on high-frequency tasks. The complexity of time series and the low-frequency preference limit the utility of sequence encoders. To address these challenges, we need to construct augmented samples from the frequency domain, thus improving the ability to accommodate events of different frequency sizes. To this end, we propose a novel Contrastive Learning with Frequency-Domain Interest Trends for Sequential Recommendation (CFIT4SRec). We treat the embedding representations of historical interactions as “images” and introduce the second-order Fourier transform to construct augmented samples. The components of different frequency sizes reflect the interest trends between attributes and their surroundings in the hidden space. We introduce three data augmentation operations to accommodate events of different frequency sizes: low-pass augmentation, high-pass augmentation, and band-stop augmentation. Extensive experiments on four public benchmark datasets demonstrate the superiority of CFIT4SRec over the state-of-the-art baselines. The implementation code is available at https://github.com/zhangyichi1Z/CFIT4SRec.
Join the Conversation
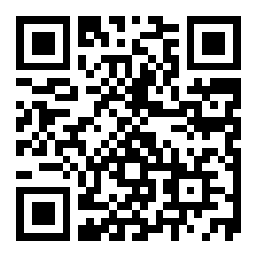