Live Session
Thursday Posters
Main Track
CR-SoRec: BERT driven Consistency Regularization for Social Recommendation
Tushar Prakash (Sony Research India), Raksha Jalan (Sony Research india), Brijraj Singh (Sony Research india) and Naoyuki Onoe (Sony).
Abstract
In the real world, when we seek our friends’ opinions on various items or events, we request verbal social recommendations. It has been observed that we often turn to our friends for recommendations on a daily basis. The emergence of online social platforms has enabled users to share their opinion with their social connections. Therefore, we should consider users’ social connections to enhance online recommendation performance. The social recommendation aims to fuse social links with user-item interactions to offer more relevant recommendations. Several efforts have been made to develop an effective social recommendation system. However, there are two significant limitations to current methods: First, they haven’t thoroughly explored the intricate relationships between the diverse influences of neighbours on users’ preferences. Second, existing models are vulnerable to overfitting due to the relatively low number of user-item interaction records in the interaction space. For the aforementioned problems, this paper offers a novel framework called CR-SoRec, an effective recommendation model based on BERT and Consistency Regularization. This model incorporates Bidirectional Encoder Representations from Transformer(BERT) to learn bidirectional context-aware user and item embeddings with neighbourhood sampling. The neighbourhood Sampling technique samples the most influential neighbours for all the users/ items. Further, to effectively use the available user-item interaction data and social ties, we leverage diverse perspectives via consistency regularisation to harness the underlying information. The main objective of our model is to predict the next item that a user would interact with based on its interaction behaviour and social connections. Experimental results show that our model defines a new state-of-the-art on various datasets and outperforms previous work by a significant margin. Extensive experiments are conducted to analyse the method. We release the source code of our model at https://anonymous.4open.science/r/CR-SoRec-68F4
Join the Conversation
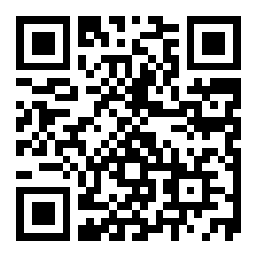