Live Session
Session 5: Sequential Recommendation 1
Research
Distribution-based Learnable Filters with Side Information for Sequential Recommendation
Haibo Liu (School of Cyber Security and Computer, HeBei university), Zhixiang Deng (School of Cyber Security and Computer, HeBei university), Liang Wang (School of Cyber Security and Computer, HeBei university), Jinjia Peng (School of Cyber Security and Computer, HeBei university) and Shi Feng (School of Computer Science & Engineering, Northeastern University)
Abstract
Sequential Recommendation aims to predict the next item by mining out the dynamic preference from user previous interactions. However, most methods represent each item as a single fixed vector, which is incapable of capturing the uncertainty of item-item transitions that result from time-dependent and multifarious interests of users. Besides, they fail to effectively exploit side information that helps to better express user preferences. At last, the noise in user’s access sequence, which is due to accidental clicks, can interfere with the next item prediction and lead to lower recommendation performance. To deal with these issues, we propose DLFS-Rec, a novel model that combines Distribution-based Learnable Filters with Side information for sequential Recommendation. Specifically, items and their side information are represented by stochastic Gaussian distribution, which is described by mean and covariance embeddings, and then the corresponding embeddings are fused to generate a final representation for each item. To attenuate noise, stacked learnable filter layers are applied to smooth the fused embeddings. The similarities between the distributions inferred from the last filter layer and candidates are measured by 2-Wasserstein distance for generating recommendation list. Extensive experiments on four public real-world datasets demonstrate the superiority of the proposed model over state-of-the-art baselines, especially on cold start users and items.
Join the Conversation
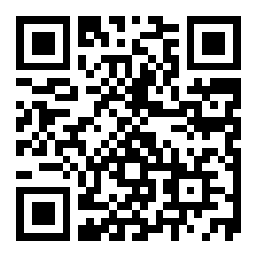