Live Session
Session 13: Side Information, Items structure and Relations
Research
Full Index Deep Retrieval: End-to-End User and Item Structures for Cold-start and Long-tail Item Recommendation
Zhen Gong (Shanghai Jiao Tong University), Xin Wu (Bytedance Inc.), Lei Chen (Bytedance Inc.), Zhenzhe Zheng (Shanghai Jiao Tong University), Shengjie Wang (Bytedance Inc.), Anran Xu (Shanghai Jiao Tong University), Chong Wang (Bytedance Inc.) and Fan Wu (Shanghai Jiao Tong University)
Abstract
End-to-end retrieval models, such as Tree-based Models (TDM) and Deep Retrieval (DR), have attracted a lot of attention, but they are flawed in cold-start and long-tail item recommendation scenarios. Specifically, DR learns a compact indexing structure, enabling efficient and accurate retrieval for large recommendation systems. However, it is discovered that DR largely fails on retrieving cold-start and long-tail items. This is because DR only utilizes user-item interaction data, which is rare and often noisy for cold-start and long-tail items. And the end-to-end retrieval models are unable to make use of the rich item content features. To address this issue while maintaining the efficiency of DR indexing structure, we propose Full Index Deep Retrieval (FIDR) that learns indices for the full corpus items, including cold-start and long-tail items. In addition to the original structure in DR (called User Structure in FIDR) that learns with user-item interaction data (e.g., clicks), we add an Item Structure to embed items directly based on item content features (e.g., categories). With joint efforts of User Structure and Item Structure, FIDR makes cold-start items retrievable and also improves the recommendation quality of long-tail items. To our best knowledge, FIDR is the first to solve the cold-start and long-tail recommendation problem for the end-to-end retrieval models. Through extensive experiments on three real-world datasets, we demonstrate that FIDR can effectively recommend cold-start and long-tail items and largely promote overall recommendation performance without sacrificing inference efficiency. According to the experiments, the recall of FIDR is improved by 8.8% ~ 11.9%, while the inference of FIDR is as efficient as DR.
Join the Conversation
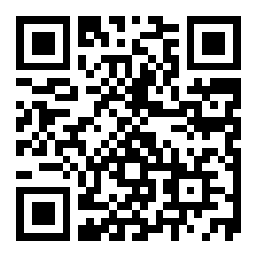