Live Session
Session 7: Interactive Recommendation 1
Research
Goal-Oriented Multi-Modal Interactive Recommendation with Verbal and Non-Verbal Relevance Feedback
Yaxiong Wu (University of Glasgow), Craig Macdonald (University of Glasgow) and Iadh Ounis (University of Glasgow
Abstract
Interactive recommendation enables users to provide verbal and non-verbal relevance feedback (such as natural-language critiques and likes/dislikes) when viewing a ranked list of recommendations (such as images of fashion products) to guide the recommender system towards their desired items (i.e. goals) across multiple interaction turns. The multi-modal interactive recommendation (MMIR) task has been successfully formulated with deep reinforcement learning (DRL) algorithms by simulating the interactions between an environment (i.e. a user) and an agent (i.e. a recommender system). However, it is typically challenging and unstable to optimise the agent to improve the recommendation quality associated with implicit learning of multi-modal representations in an end-to-end fashion in DRL. This is known as the coupling of policy optimisation and representation learning. To address this coupling issue, we propose a novel goal-oriented multi-modal interactive recommendation model (GOMMIR) that uses both verbal and non-verbal relevance feedback to effectively incorporate the users’ preferences over time. Specifically, our GOMMIR model employs a multi-task learning approach to explicitly learn the multi-modal representations using a multi-modal composition network when optimising the recommendation agent. Moreover, we formulate the MMIR task using goal-oriented reinforcement learning and enhance the optimisation objective by leveraging non-verbal relevance feedback for hard negative sampling and providing extra goal-oriented rewards to effectively optimise the recommendation agent. Following previous work, we train and evaluate our GOMMIR model by using user simulators that can generate natural-language feedback about the recommendations as a surrogate for real human users. Experiments conducted on four well-known fashion datasets demonstrate that our proposed GOMMIR model yields significant improvements in comparison to the existing state-of-the-art baseline models
Join the Conversation
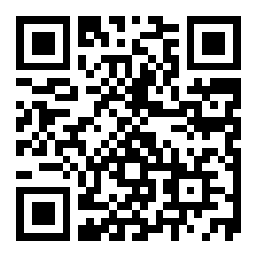