Live Session
Session 2: Click-Through Rate prediction
Research
Gradient Matching for Categorical Data Distillation in CTR Prediction
Cheng Wang (School of Cyber Science and Engineering, Huazhong University of Science and Technology, Wuhan), Jiacheng Sun (Huawei Noah’s Ark Lab), Zhenhua Dong (Huawei Noah’s Ark Lab), Ruixuan Li (School of Computer Science and Technology, Huazhong University of Science and Technology, Wuhan) and Rui Zhang (ruizhang.info)
Abstract
The cost of hardware and energy consumption on training a click-through rate (CTR) model is highly prohibitive. A recent promising direction for reducing such costs is data distillation with gradient matching, which aims to synthesize a small distilled dataset to guide the model to a similar parameter space as those trained on real data. However, there are two main challenges to implementing such a method in the recommendation field: (1) The categorical recommended data are high dimensional and sparse one- or multi-hot data which will block the gradient flow, causing back propagation data distillation invalid. (2) The data distillation process with gradient matching is computationally expensive due to the bi-level optimization. To this end, we investigate efficient data distillation tailored for recommendation data with plenty of side information where we formulate the discrete data to the dense and continuous data format. Then, we further introduce a one-step gradient matching scheme, which performs gradient matching for only a single step to overcome the inefficient training process. The overall proposed method is called Categorical data distillation with Gradient Matching (CGM), which is capable of distilling a large dataset into a small of informative synthetic data for training CTR models from scratch. Experimental results show that our proposed method not only outperforms the state-of-the-art coreset selection and data distillation methods but also has remarkable cross-architecture performance. Moreover, we explore the application of CGM on continual updating and mitigate the effect of different random seeds on the training results.
Join the Conversation
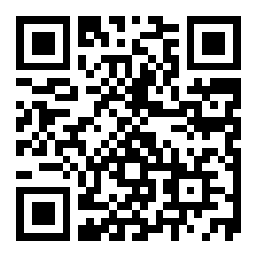