Live Session
Friday Posters
Main Track
LLM4Rec: Large Language Models for Recommendation via A Lightweight Tuning Framework
Keqin Bao (University of Science and Technology in China), Jizhi Zhang (University of Science and Technology in China), Yang Zhang (University of Science and Technology of China), Wenjie Wang (National University of Singapore), Fuli Feng (University of Science and Technology in China) and Xiangnan He (University of Science and Technology of China).
Abstract
The impressive performance of Large Language Models (LLMs) across various fields has encouraged researchers to investigate their potential in recommendation tasks. To harness the LLMs’ extensive knowledge and powerful generalization abilities, initial efforts have tried to design instructions for recommendation tasks through In-context Learning. However, the recommendation performance of LLMs remains limited due to (i) significant differences between LLMs’ language-related pre-training tasks and recommendation tasks, and (ii) inadequate recommendation data during the LLMs’ pre-training. To fill the gap, we consider further tuning LLMs for recommendation tasks. To this end, we propose a lightweight tuning framework for LLMs-based recommendation, namely LLM4Rec, which constructs the recommendation data as tuning samples and utilizes LoRA for lightweight tuning. We conduct experiments on two datasets, validating that LLM4Rec is highly efficient w.r.t. computing costs (e.g., a single RTX 3090 is sufficient for tuning LLaMA-7B), and meanwhile, it can significantly enhance the recommendation capabilities of LLMs in the movie and book domains, even with limited tuning samples (< 100 samples). Furthermore, LLM4Rec exhibits strong generalization ability in cross-domain recommendation. Our code and data are available at https://anonymous.4open.science/r/LLM4rec.
Join the Conversation
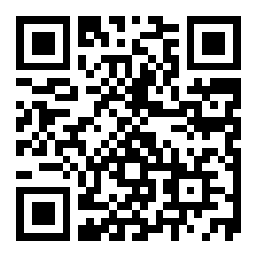