Live Session
Thursday Posters
Main Track
Private Matrix Factorization with Public Item Features
Mihaela Curmei (University of California, Berkeley), Walid Krichene (Google Research) and Li Zhang (Google Research).
Abstract
We consider the problem of training private recommendation models with access to public item features. Training with Differential Privacy (DP) offers strong privacy guarantees, at the expense of loss in recommendation quality. We show that incorporating public item features during training can help mitigate this loss in quality. We propose a general approach based on collective matrix factorization, that works by simultaneously factorizing two matrices: the user feedback matrix (representing sensitive data) and an item feature matrix that encodes publicly available (non-sensitive) item information.
The method is conceptually simple, easy to tune, and highly scalable. It can be applied to different types of public data, including: (1) categorical item features; (2) item-item similarities learned from public sources; and (3) publicly available user feedback.
Evaluating our method on a standard DP recommendation benchmark, we find that using public item features significantly narrows the quality gap between the private models and their non-private counterpart. As privacy constraints become more stringent, the increased reliance on public side features leads to recommendations becoming more depersonalized, resulting in a smooth transition from collaborative filtering to item-based contextual recommendations.
Join the Conversation
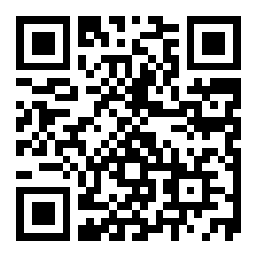