Live Session
Friday Posters
Main Track
Progressive Horizon Learning: Adaptive Long Term Optimization for Personalized Recommendation
Congrui Yi (Amazon), David Zumwalt (Amazon), Zijian Ni (Amazon) and Shreya Chakrabarti (Amazon).
Abstract
As B2C companies such as Amazon, Netflix, Spotify scale, personalized recommender systems are often needed to further drive long term business growth in acquisition, engagement, and retention of customers. However, long-term metrics associated with these goals can require several months to mature. Additionally, deep personalization also demands a large volume of training data that take a long time to collect. These factors incur substantial lead time for training a model to optimize a long-term metric. Before such model is deployed, a recommender system has to rely on a simple policy (e.g. random) to collect customer feedback data for training, inflicting high opportunity cost and delaying optimization of the target metric. Besides, as customer preferences can shift over time, a large temporal gap between inputs and outcome poses a high risk of data staleness and suboptimal learning. Existing approaches involve various compromises. For instance, contextual bandits often optimize short-term surrogate metrics with simple model structure, which can be suboptimal in the long run, while Reinforcement Learning approaches rely on an abundance of historical data for offline training, which essentially means long lead time before deployment. To address these problems, we propose Progressive Horizon Learning Recommender (PHLRec), a personalized model that can progressively learn metric patterns and adaptively evolve from short- to long-term optimization over time. Through simulations and real data experiments, we demonstrated that PHLRec outperforms competing methods, achieving optimality in both deployment speed and long-term metric performances.
Join the Conversation
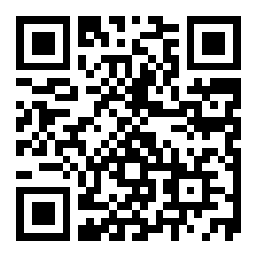