Live Session
Session 16: Multimedia Recommendation
Reproducibility
Reproducibility Analysis of Recommender Systems relying on Visual Features: traps, pitfalls, and countermeasures
Pasquale Lops (University of Bari), Elio Musacchio (Università degli Studi di Bari Aldo Moro), Cataldo Musto (Dipartimento di Informatica – University of Bari), Marco Polignano (Università degli Studi di Bari Aldo Moro), Antonio Silletti (Dipartimento di Informatica – University of Bari) and Giovanni Semeraro (Dipartimento di Informatica – University of Bari)
Abstract
Reproducibility is an important requirement for scientific progress, and the lack of reproducibility for a large amount of published research can hinder the progress over the state-of-the-art. This concerns several research areas, and recommender systems are witnessing the same reproducibility crisis. Even solid works published at prestigious venues might not be reproducible for several reasons: data might not be public, source code for recommendation algorithms might not be available or well documented, and evaluation metrics might be computed using parameters not explicitly provided. In addition, recommendation pipelines are becoming increasingly complex due to the use of deep neural architectures or representations for multimodal side information involving text, images, audio, or video. This makes the reproducibility of experiments even more challenging. In this work, we describe an extension of an already existing open-source recommendation framework, called ClayRS, with the aim of providing the foundation for future reproducibility of recommendation processes involving images as side information. This extension, called ClayRS Can See, is the starting point for reproducing state-of-the-art recommendation algorithms exploiting images. We have provided our implementation of one of these algorithms, namely VBPR – Visual Bayesian Personalized Ranking from Implicit Feedback, and we have discussed all the issues related to the reproducibility of the study to deeply understand the main traps and pitfalls, along with solutions to deal with such complex environments. We conclude the work by proposing a checklist for recommender systems reproducibility as a guide for the research community.
Join the Conversation
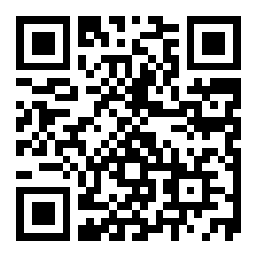