Live Session
Session 4: Trustworthy Recommendation
Research
Towards Robust Fairness-aware Recommendation
Hao Yang (Gaoling School of Artificial Intelligence, Renmin University of China, Beijing, China), Zhining Liu (Ant Group), Zeyu Zhang (Gaoling School of Artificial Intelligence, Renmin University of China, Beijing, China), Chenyi Zhuang (Ant Group) and Xu Chen (Gaoling School of Artificial Intelligence, Renmin University of China, Beijing, China)
Abstract
Due to the progressive advancement of trustworthy machine learning algorithms, fairness in recommender systems is attracting increasing attention and is often considered from the perspective of users. Conventional fairness-aware recommendation models make the assumption that user preferences remain the same between the training set and the testing set. However, this assumption is disagreed with reality, where user preference can shift in the testing set due to the natural spatial or temporal heterogeneity. It is concerning that conventional fairness-aware models may be unaware of such distribution shifts, leading to a sharp decline in the model performance. To address the distribution shift problem, we propose a robust fairness-aware recommendation framework based on Distributionally Robust Optimization (DRO) technique. In specific, we assign learnable weights for each sample to approximate the distributions that leads to the worst-case model performance, and then optimize the fairness-aware recommendation model to improve the worst-case performance in terms of both fairness and recommendation accuracy. By iteratively updating the weights and the model parameter, our framework can be robust to unseen testing sets. To ease the learning difficulty of DRO, we use a hard clustering technique to reduce the number of learnable sample weights. To optimize our framework in a full differentiable manner, we soften the above clustering strategy. Empirically, we conduct extensive experiments based on four real-world datasets to verify the effectiveness of our proposed framework. For benefiting the research community, we have released our project at https://anonyrobfair.github.io/.
Join the Conversation
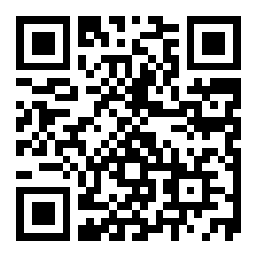