Live Session
Session 16: Multimedia Recommendation
Research
Towards the Understanding and Modeling of Passive-Negative Feedback in Sequential Short-video Recommendation
Yunzhu Pan (UESTC), Chen Gao (Tsinghua University), Yang Song (Kuaishou Inc.), Kun Gai (Unaffiliated), Depeng Jin (Department of Electronic Engineering, Tsinghua University) and Yong Li (Tsinghua University)
Abstract
Sequential recommendation is one of the most important tasks in recommender systems, which aims to recommend the next interacted item with historical behaviors as input. Traditional sequential recommendation always mainly considers the collected positive feedback such as click, purchase, etc. However, in short-video platforms such as TikTok, video viewing behavior may not always represent positive feedback. Specifically, the videos are played automatically, and users passively receive the recommended videos. In this new scenario, users passively express negative feedback by skipping over videos they do not like, which provides valuable information about their preferences. Different from the negative feedback studied in traditional recommender systems, this passive-negative feedback can reflect users’ interests and serve as an important supervision signal in extracting users’ preferences. Therefore, it is essential to carefully design and utilize it in this novel recommendation scenario. In this work, we first conduct analyses based on a large-scale real-world short-video behavior dataset and illustrate the significance of leveraging passive feedback. We then propose a novel method that deploys the sub-interest encoder, which incorporates positive feedback and passive-negative feedback as supervision signals to learn the user’s current active sub-interest. Moreover, we introduce an adaptive fusion layer to integrate various sub-interests effectively. To enhance the robustness of our model, we then introduce a multi-task learning module to simultaneously optimize two kinds of feedback – passive-negative feedback and traditional randomly-sampled negative feedback. The experiments on two large-scale datasets verify that the proposed method can significantly outperform state-of-the-art approaches. The codes and collected datasets are anonymously released at https:// anonymous.4open.science/ r/ SINE-2047/ to benefit the community.
Join the Conversation
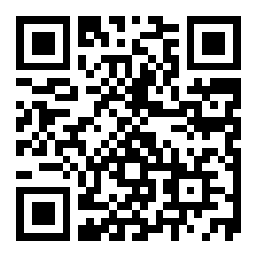